National Institute of Health Postdoctoral Scholar - Computational Models of Human Comprehension
Stanford University - 1990
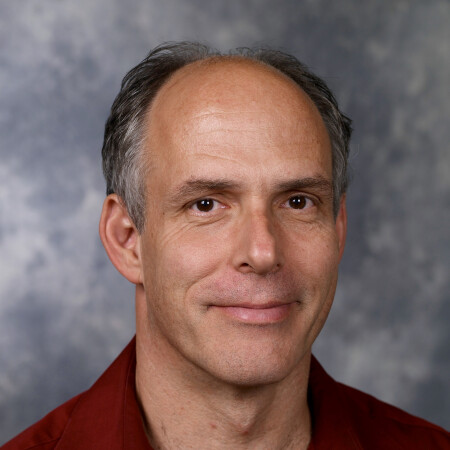
Richard Golden
Participating Faculty Member in Electrical Engineering and Computer Science
Professor
Program Head, Applied Cognition and Neuroscience MS
Program Head, Cognitive Science BS
Research Interests: Computational psychometrics, learning sciences, and statistical machine learning.
972-883-2423
GR 4.814
COINS Lab
Personal Home Page
Coins Lab Twitter
Curriculum Vitae
ORCID
Currently accepting undergraduate and graduate students
Professional Preparation
Andrew Mellon Postdoctoral Scholar - Computational Models of Human Comprehension
University of Pittsburgh LRDC - 1988
University of Pittsburgh LRDC - 1988
Ph.D. - Experimental Psychology (Computational Cognitive Science)
Brown University - 1987
Brown University - 1987
M.S. - Electrical Engineering (Statistical Pattern Recognition)
Brown University - 1986
Brown University - 1986
B.S. - Electrical Engineering (Communication Systems) and Psychology
University of California at San Diego - 1982
University of California at San Diego - 1982
Research Areas
Computational Psychometrics
The current research focus of my lab lies in the multidisciplinary field of Computational Psychometrics. Computational Psychometrics integrates methods of psychometrics, learning science, usability engineering, mathematical statistics, and statistical machine learning. In particular, the focus of my research lab is concerned with the development of new mathematical tools and models that quantitatively characterize what latent skills are learned by students and how those latent skills are learned.This new research effort builds upon prior work including: theoretical investigations of the asymptotic behavior of machine learning algorithms (Golden, 2018, 2020), methods for selecting the most appropriate model (Golden, 2003; Golden et al. 2015; Golden, Nandy, and Patel, 2019; Golden, 2020), the detection of model misspecification (Golden et al. 2013, 2016), and estimation and inference in possibly misspecified models when both latent variables and missing data are present (Golden, 1995; Golden, 2003; Golden, Henley, White, and Kashner 2019; Golden, 2020). Many of these prior research threads are summarized in my new book Statistical Machine Learning: A unified framework (Golden, 2020) which was published in the Texts in Statistical Science Series (CRC Press).
Statistical Machine Learning
Dr. Golden's long-term research interests in Artificial Neural Networks and Statistical Machine Learning have been concerned with the development of a formal unified probabilistic framework for interpreting inference and learning processes in machine learning algorithms (Golden, 1988a; Golden, 1988b; Golden, 1988c; Rumelhart et al., 1995; Golden 1996a; Golden, 1996b; Golden, 1997) as well as convergence analyses of machine learning algorithms (Golden, 1986; Golden, 1993; Golden, 2018). Dr. Golden's new graduate computer science textbook Statistical Machine Learning: A unified framework (Golden, 2020) introduces and reviews such methods.Computational Cognitive Science
Dr. Golden's long-term research interests in Computational Cognitive Science and Mathematical Psychology have focused upon the development and evaluation of formal models and theories of human comprehension. Golden (1986) explored how bottom-up and top-down processing mechanisms might be learned from experience using a computational artificial neural network model. Golden and Rumelhart (1993), Golden et al. (1994), and Golden (1997) propose computational models of text recall and summarization. Golden (1994), Golden (1998), Jaynes and Golden (2003), and Goldman and Golden (2006) analyzed temporal structure in human story recall and summarization data using the Knowledge Digraph Contribution Analysis (KDC) analysis developed by Golden (1994) and Golden (1998). KDC analysis is based upon a psychometric model for generating recall and summarization data whose parameters represent the influence of different types of knowledge schemata represented as directed graphs. Durbin, Earwood, and Golden (2000) and Ghiasinejad and Golden (2013) developed and evaluated a hidden Markov model designed to interact with human coders to assist in the coding of free response data in text recall and text summarization experiments.Publications
Making causal inferences about treatment effect sizes from observational datasets 2020 - Journal Article
Consequences of model misspecification for maximum likelihood estimation with missing data 2019 - Journal Article
Response to letter regarding "a systematic approach to subgroup analyses in a smoking cessation trial" 2016 - Journal Article
Awards
Invited Speaker for "Robotics, Artificial Intelligence, and Machine Learning: 2020 Challenges for Urological Research Symposium Series" - American Urological Association [2020]
Secretary-Treasurer Service Award (15 years) - Society for Mathematical Psychology [2017]
Invited Speaker for "New Strategies to Solve Analytic Challenges in Health Services Research" Workshop - Veteran's Administration Health Services Research and Development [2016]
Keynote Speaker - 34th Annual Meeting of the Society for Mathematical Psychology [2001]
IEEE Senior Member - Institute of Electrical and Electronics Engineers [1999]
Faculty Development Award to Study Econometrics with Professor Halbert L. White - University of Texas at Dallas [1998]
Keynote Speaker - 2nd Joint Mexico-US International Conference on Neural Networks and Neurocontrol [1997]
Appointments
Professor of Cognitive Science
University of Texas at Dallas [2004–Present]
University of Texas at Dallas [2004–Present]
Program Head, Applied Cognition and Neuroscience Graduate Program
University of Texas at Dallas [2000–Present]
University of Texas at Dallas [2000–Present]
Program Head, Undergraduate Cognitive Science Program
University of Texas at Dallas [2000–Present]
University of Texas at Dallas [2000–Present]
Participating Faculty Member in Electrical Engineering
University of Texas at Dallas [1999–Present]
University of Texas at Dallas [1999–Present]
Associate Professor of Cognitive Science
University of Texas at Dallas [1996–2004]
University of Texas at Dallas [1996–2004]
Assistant Professor of Cognitive Science
University of Texas at Dallas [1990–1996]
University of Texas at Dallas [1990–1996]
Projects
Supporting Classroom Learning Outcome Assessment using a Longitudinal Higher-Order Cognitive Diagnostic Model
2020/07 This project develops advanced statistical machine learning measurement methodologies specifically designed to support low-stakes classroom instruction by the typical classroom instructor for in person, hybrid, and online learning environments. A key feature of the proposed project is that the psychometric measurement tools estimate student mastery of instructor-specified course learning outcomes at various assessment time periods throughout the semester while simultaneously integrating information across all assessment time periods.Additional Information
Editorial and Governing Boards
Editorial Board Member (Action Editor)- 1996-2011, Journal of Mathematical Psychology
- 1995-2006, Neural Networks
- 2001-2004, International Journal of Applied Intelligence
- 1999-2004, Neural Processing Letters.
- 2002-2017 Society for Mathematical Psychology (Secretary-Treasurer)
- 1996-2004 Society for Text and Discourse which is a member of the International Alliance to Advance Learning in the Digital Era (IAALE)
Patents
- Graphical User Interface for Automatic Coding of Free Response Data using Hidden Markov Model Methodology. Golden, R.M., Earwood, J., Durbin, M. A. Patent No. US 7,188,064. Assignee: Board of Regents, The University of Texas System, Austin, TX. April 12, 2002.
- Adaptive Multiple Access Interference Suppression. Dowling, E. M., Jani, U. G., Wang, Z., Golden, R. M. U.S. Patent No. US 6,700,923 B1. Assignee: Board of Regents, The University of Texas System, Austin, TX. March 2, 2004.
- Smart Antenna Multiuser Detector. Dowling, E. M., Jani, U., Golden, R. M., Wang, Z.U.S. Patent No. US 6,782,036 B1. Assignee: Board of Regents, The University of Texas System, Austin, TX. August 24, 2004.